The insurance industry is at a technological crossroads. Today, the AI opportunity in insurance is broad, and AI promises significant improvements for the cognitive heavy lifting necessary to assess risk, process claims and detect fraud. Here, generative AI technologies are set to redefine the competitive landscape.
However, the mere potential of these technologies does not render them immune to the significant challenges that insurers face when trying to harness the power of any AI technology:
- Acquiring and retaining the specialized (and scarce) skills required to develop and deploy generative AI systems.
- Planning for the significant upfront investments, ongoing maintenance costs and skilled technology resources needed.
- Changes to data governance approaches and regulatory compliance considerations.
- Ensuring model accuracy, fidelity and trustworthiness.
Herein lies the crux of the issue: can these systems be trusted to deliver the right outcomes consistently, without prejudice and without hallucination? The answer is yes. In this post, we look at how domain-trained language models and advanced fine-tuning techniques offer insurers a safe, cost-effective way to access the generative AI tools they need.
A Large Language Model for Insurance
The Enterprise Language Model for Insurance (ELMI) provides insurers with the capabilities of generative AI and LLMs safely, securely, cost-effectively and with the domain-specific knowledge that insurance processes require.
Unlike general LLMs, ELMI is trained with a deep understanding of the insurance domain. This allows insurers to leverage capabilities like:
Medical Record Summaries
Medical record summarization helps claims and underwriting teams condense complex medical information into concise summaries. This enables teams to quickly assess the medical history and current health status of applicants or claimants. Summarization also highlights key information, such as diagnoses, treatments and outcomes, helping teams make more informed decisions about claims and underwriting.
By automating the review process, medical record summarization allows for complete coverage of submitted medical records, reduces skilled labor costs and enhances the overall quality of data-driven decision-making for insurance teams.
Automated Claims Data Extraction and Enrichment
Claim data extraction automates the process of extracting data from various sources, reducing manual data entry and improving accuracy. Extracted data can be normalized and augmented against ICD, CPT and other standards. This automation speeds up claims processing, leading to faster settlements and improved customer satisfaction.
Natural Language Q&A
Claims professionals can ask questions in everyday language (“What is the accident date?”) and receive relevant answers (“The accident occurred on February 22, 2024.”) extracted from and traceable to the original claim files. This improves efficiency by reducing the time spent manually searching through documents.
ELMI is designed to make it easy to access reusable, secure and real-world-tested functionality specifically built for insurance companies but without the cost or complexity involved with training an LLM.
ELMI can read, understand and extract essential data from medical documentation, accident reports and claims submissions, score risk engineering reports, triage claims based on urgency and severity, classify records by type, and generate summaries.
The advantage of an AI model like ELMI is that it’s already trained on the knowledge, language and scenarios specific to the insurance domain. ELMI is not just a language model, but a knowledge model that contains pre-built knowledge on the insurance domain and a framework that allows it to accurately structure any new knowledge that the model acquires.
While there will always be a need to incorporate new data or knowledge over time or to fine tune a model’s results to ensure they are aligned with your business requirements, this provides a much more accurate and cost-effective starting point from which to begin.
For example, every insurance company has their own business rules for processing documents like medical records or for executing certain tasks. With ELMI, specific terminology and ways of handling information can be trained into the model.
Comparing Performance: Benchmarks
For insurers who are evaluating different LLM-based AI solutions, benchmarks offer a standardized way to compare important performance indicators.
We use benchmark testing to compare ELMI’s performance with those of base LLMs, such as GPT, Llama and others. The results below show how each model performed on key insurance tasks like the ones listed above in terms of three key indicators:
- Answer Similarity – closeness of response to predetermined output result.
- Truthfulness – model strength against hallucinations and other possible results not coming from context.
- Formal Correctness – model ability to generate formally correct results for integration in application workflows.
All benchmark test cases are tasks that request answers from the provided input text, not from the general knowledge model. This makes it possible to safely constrain the model in order to properly analyze documents and provide information from those documents so that ELMI can be used for insurance document intelligent analysis. This limits the chance of hallucinations where models can return out-of-context responses that are not related to the content being analyzed.
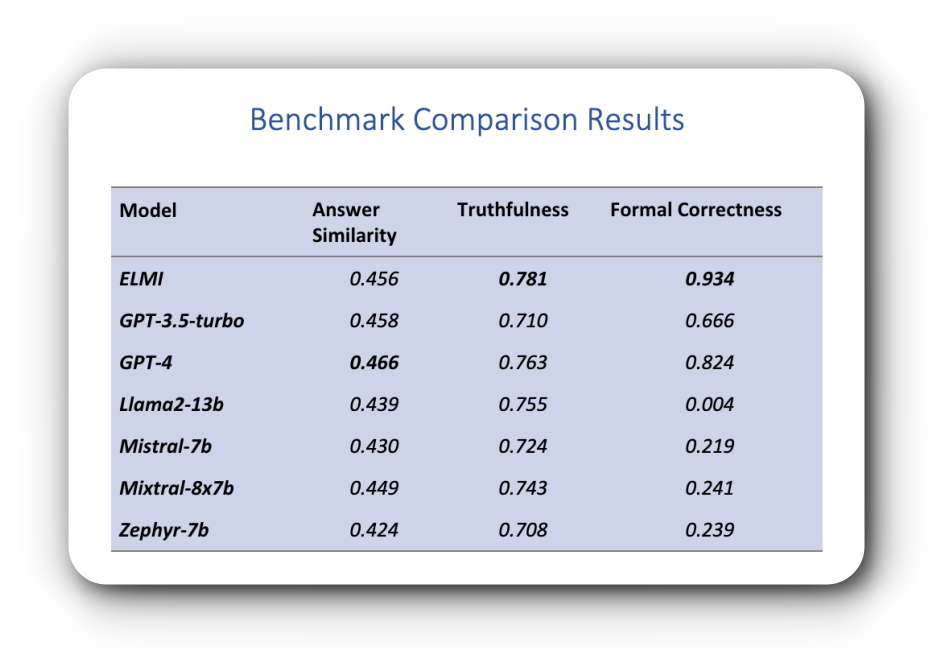
Performance Highlights
These results are thanks to the work of the expert.ai team to make LLMs and generative AI more effective and efficient for practical, enterprise-grade applications.
- ELMI shows accuracy that is on par with GPT-3.5-turbo and close to GPT-4, with a ~24.3% reduction on the chance of hallucinations with respect to GPT-3.5-turbo and a ~7.4% with respect to GPT-4, thanks to instruct based fine-tuning.
- Because ELMI uses a knowledge-based Retrieval Augmented Generation approach for more precise inputs and uses advanced LLM processing techniques for easier to understand response outputs, the results are more truthful and provide complete traceability to the source material.
- ELMI also shows a huge improvement compared to other models for Formal Correctness scoring, which results in cost savings and subsequent ease of application integration.
- In terms of speed, ELMI demonstrates a large efficiency boost thanks to expert.ai innovations, such as the use of Fast Vocabulary Transfer (FVT) and Multi-Word Tokenization (MWT), which shows that we can achieve 1.8x faster performance without sacrificing quality. Meta (Facebook) is exploiting the same expert.ai innovations for their upcoming LLMs: “We confirm that FVT leads to noticeable improvement on all downstream tasks,” as cited in a recent paper.
- ELMI can run on a single GPU machine, with consequential cost savings (over typical LLMs) on significant volumes of processed documents.
Conclusion
While LLMs are extremely powerful, they come with considerable precision, bias and cost challenges that cannot be ignored. By combining insurance-specific knowledge models with LLMs and utilizing cutting-edge LLM techniques, ELMI not only mitigates these issues but also saves time and costs. That’s why ELMI stands out as a unique alternative for insurers who want to leverage GenAI capabilities: it uniquely tackles insurance challenges and provides a real-world solution that insurers can adopt today.